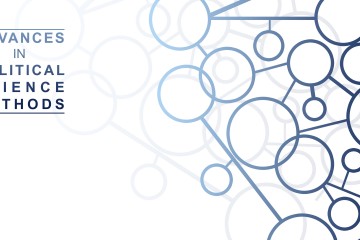
Estimating the Effect of Feature Selection in Computational Text Analysis
Below, I discuss and analyse pre-processing decisions in relation to an often-used application of text analysis: scaling. Here, I’ll be using a new tool, called preText (for R statistical software), to investigate the potential effect of different pre-processing options on our estimates. Replication material for this post may be found on my GitHub page. Feature Selection and Scaling Scaling algorithms rely on the bag-of-words (BoW) assumption, i.e. the idea that we can reduce text to individual words and sample them independently from a “bag” and still get some meaningful insights from the relative distribution of words across a corpus. For the demonstration below, I’ll be using the same selection of campaign speeches from one of my earlier blog posts, in which I used a …
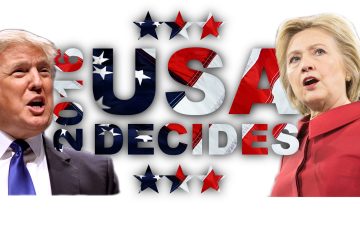
Words that matter: What text analysis can tell us about the third presidential debate
The final Presidential debate of 2016 was as heated as the previous two—well demonstrated by the following name-calling exchange: CLINTON: …[Putin would] rather have a puppet as president of the United States. TRUMP: No puppet. No puppet. CLINTON: And it’s pretty clear… TRUMP: You’re the puppet! CLINTON: It’s pretty clear you won’t admit … TRUMP: No, you’re the puppet. It is easy to form our opinions of the debate and on the differences between the Presidential candidates on excerpts like this and memorable one-liners. But are small extracts representative of the debate as a whole? Moreover, how can we objectively analyse what was said, who got to say the most, and how the candidates differed in their responses? One approach is …