Big data, computer science, experimental methods, and computational text analysis are part of an ever-growing range of methods embraced by political science. As we expand our methodological horizons, the nature, scope, and focus of the questions we investigate will change.
For example, the combination of computerised text analysis techniques and Big Data now allows us to model millions of texts in a matter of hours. This can help us to gauge political preferences, the evolution of political language, or popular sentiment.
In turn, the increased use of the experimental method has allowed political scientists to investigate a wide set of issues, including voter behaviour, public opinion, and decision-making processes.
This new series, co-hosted by the Oxford University Politics Blog and the Oxford Q-Step Centre is all about “methods”. What advances have we seen in recent years? What can we learn today that we could not a decade ago? And, what is the future of methods in political science? Find out more in our Advances in Political Science Methods series.
About Q-Step
Q-Step is a £19.5 million programme designed to promote a step-change in quantitative social science training in the UK Funded by the Nuffield Foundation, ESRC and HEFCE, Q-Step was developed as a strategic response to the shortage of quantitatively-skilled social science graduates.
Q-Step is funding fifteen universities across the UK to establish Q-Step Centres that will support the development and delivery of specialist undergraduate programmes, including new courses, work placements and pathways to postgraduate study.
The resulting expertise and resources will be shared across the higher education sector through an accompanying support programme which will also forge links with schools and employers.
Oxford is one of 15 universities to be selected nationally to host Q-Step. The Oxford Q-Step Centre is hosted by the Department of Politics and International Relations, in close co-operation with the Department of Sociology, University of Oxford.
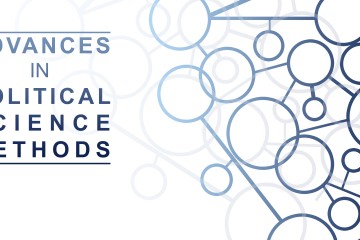
When does traditional statistics become machine learning?
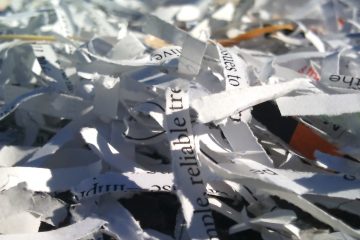
The Shorter, the Better? What it Takes to and What to Take Away from Publishing a Very Short Article
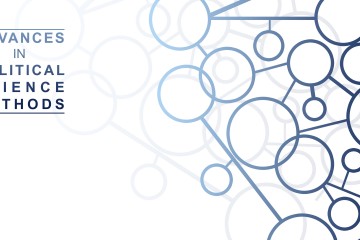
In Polls We Do Not Trust: The British Polling Experience
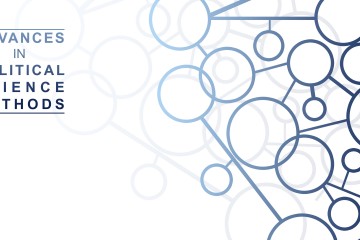
Estimating the Effect of Feature Selection in Computational Text Analysis
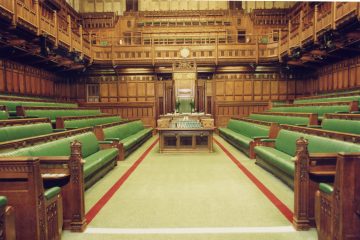
The Evolution of Legislation: a Bioinformatics Approach
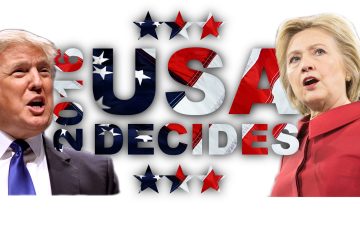
Trumped-up vs. Clintonesque: what text analysis can teach us about the US elections